
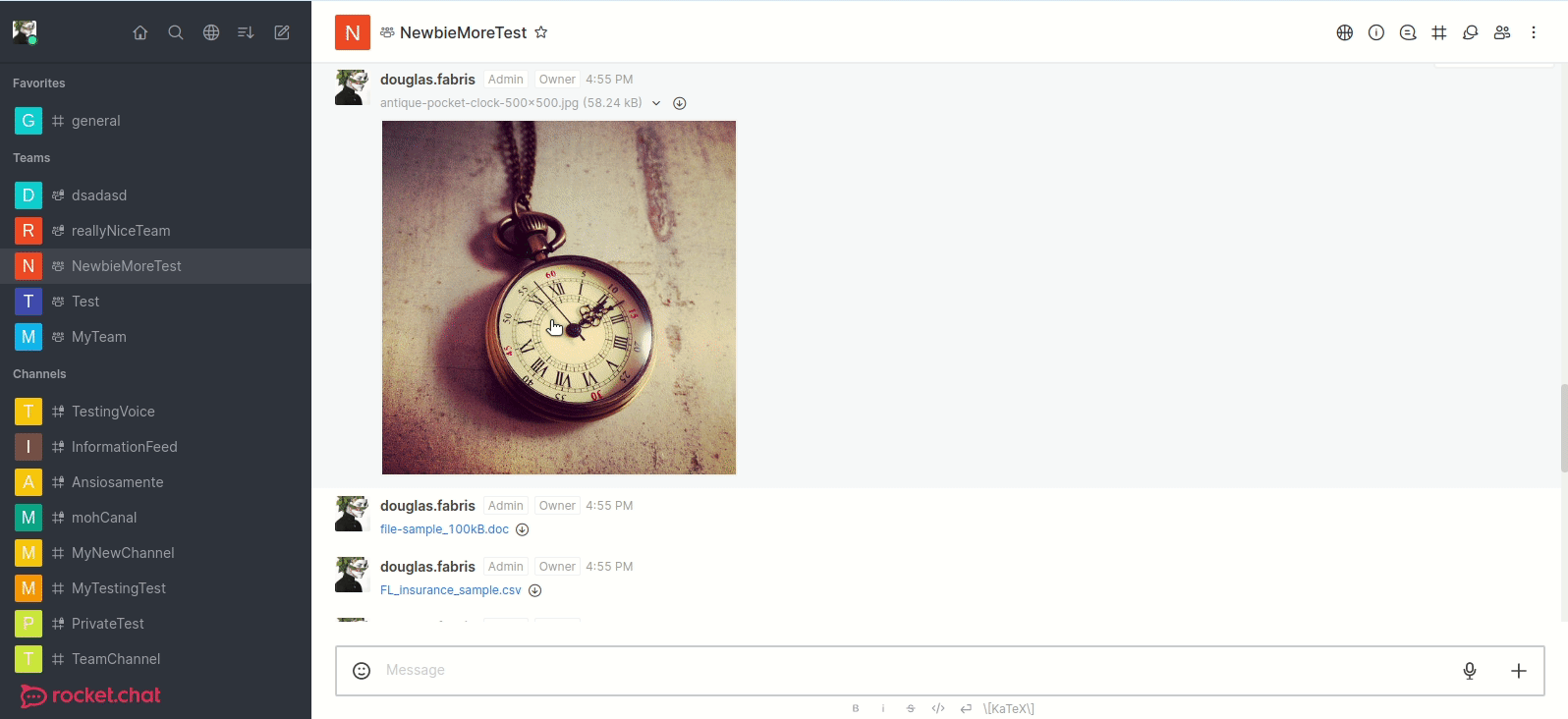
Computer Vision), as well as predictive models for various phenomena (e.g. Reinforcement Learning) and different sensor modalities (e.g.

This component intersects significantly with robotics research represented at NeurIPS, and includes several parts of real-world robotic systems such as: managing control systems (e.g. Graph Neural Networks and Machine Learning For Physics). This component intersects heavily with algorithmic research at NeurIPS, including (but not limited to) various topic areas such as: Reinforcement Learning and data-driven modeling of physical phenomena using Neural Networks (e.g. They require the integration of diverse fields and consist of three primary components, which intersect with many AI-related research topics: Self-Driving Materials Laboratories have greatly advanced the automation of material design and discovery.
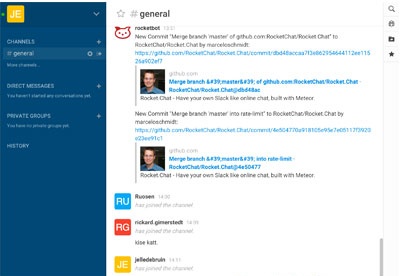
Note that even if the algorithm can learn from very few samples, we still need sufficient validation data … The training and evaluation of ML algorithms require datasets with a sufficient sample size. Specifically, high-quality synthetic data generation could be done while addressing the following major issues.ġ. Synthetic data is a promising solution to the key issues of benchmark dataset curation and publication. Hence, although ML holds strong promise in these domains, the lack of high-quality benchmark datasets creates a significant hurdle for the development of methodology and algorithms and leads to missed opportunities. They already manifest in many existing benchmarks, and also make the curation and publication of new benchmarks difficult (if not impossible) in numerous high-stakes domains, including healthcare, finance, and education. However, three prominent issues affect benchmark datasets: data scarcity, privacy, and bias. There is a general belief that the accessibility of high-quality benchmark datasets is central to the thriving of our community. Examples are abundant: CIFAR-10 for image classification, COCO for object detection, SQuAD for question answering, BookCorpus for language modelling, etc.
Rocketchat latest version how to#
Specifically, we aim to the following questions: 1) What properties are required for building trust between humans and interactive autonomous systems? How can we assess and ensure these properties without compromising the expressiveness of the models and performance of the overall systems? 2) How can we develop and deploy trustworthy autonomous agents under an efficient and trustful workflow? How should we transfer from development to deployment? 3) How to define standard metrics to quantify trustworthiness, from regulatory, theoretical, and experimental perspectives? How do we know that the …Īdvances in machine learning owe much to the public availability of high-quality benchmark datasets and the well-defined problem settings that they encapsulate. In this workshop, we bring together experts with diverse and interdisciplinary backgrounds, to build a roadmap for developing and deploying trustworthy interactive autonomous systems at scale. However, the opaque nature of deep learning models makes it difficult to decipher the decision-making process of the agents, thus preventing stakeholders from readily trusting the autonomous agents, especially for safety-critical tasks requiring physical human interactions. In particular, these agents with advanced intelligence have shown great potential in interacting and collaborating with humans (e.g., self-driving cars, industrial robot co-worker, smart homes and domestic robots). The recent advances in deep learning and artificial intelligence have equipped autonomous agents with increasing intelligence, which enables human-level performance in challenging tasks.
